AI Regulation Needs to Focus on Supercharging the Economy
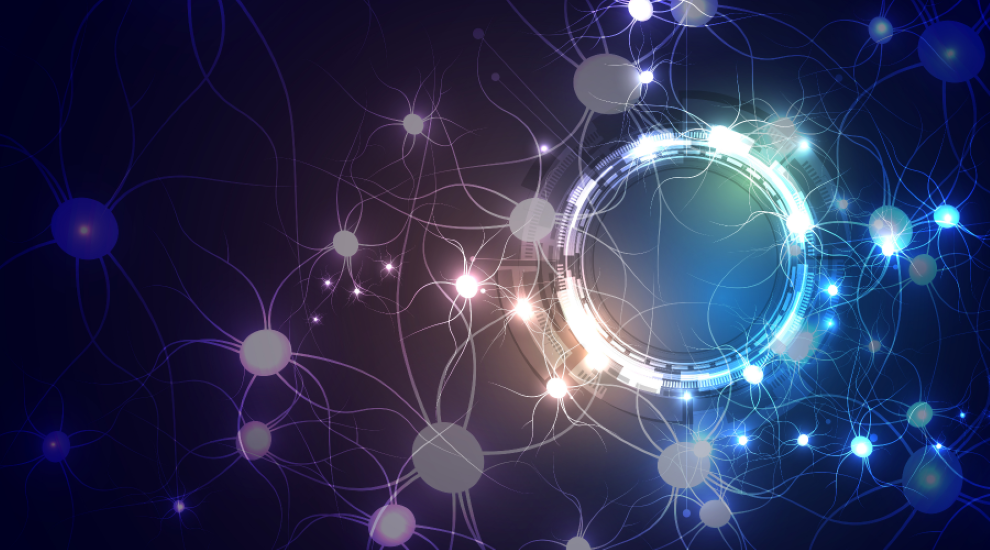


Artificial Intelligence will disrupt how we work and who we work with. The impact of earlier generations of these tools, like RPA, and more modern tools like AWS CodeWhisperer or ChatGPT are already having significant impacts, in many cases improving productivity and accelerating modernization efforts regardless of industry. It will have the same sort of impact the Internet did and still does. Some of this will be welcomed, some of this will be challenged, and some of this will be reviled. Regardless, AI is going to have long-term and lasting impacts for the foreseeable future. So much so, that years from now, people will see this period of the last few years as a transition from the social age to the AI age.
Let’s set the record straight. AI isn’t social. It’s a computational paradigm – a way of using computers in a new and novel way. AI used social in the same way that social used the Internet – both accelerated by the underlying previous technology. In AI’s case, social provided the vast datasets necessary to train the current technological leaps forward in AI. And of course, without the Internet, social would have been incredibly more constrained by region.
Why start with this context? When considering how and where to regulate AI, as some are calling for, it is incredibly important to understand that overregulating AI will create significant economic and national security implications for the nation. When combined with the fact that social was underregulated because society understood too late the more dangerous aspects of the impacts, we now stand poised to overcorrect through overregulating on a technology that may have significant long-term repercussions.
So, here’s the challenge. How might we regulate AI in a way that enhances the economic opportunities and limits national security impacts of the technology while minimizing the dangers to society? We can look at other examples in the United States to get a sense of how we might think about regulating AI in a meaningful way that adds value without unintended consequences or unacceptable risks.
AI is not nuclear or energy
CEO of OpenAI, Sam Altman gave a recent testimony in front of Congress offering some ideas on regulation that immediately harkened to how we regulate nuclear energy. His ideas included testing focused on the product or research. And while at a glance this may make sense, the reality is that this approach is the single best way to put the United States behind other global competitors. It costs significantly more money to compete in highly regulated industries – not only because of the specialized experience and technology involved, and it also requires significant interaction with the government, audits, and reviews. It has taken decades to put new ideas forward in nuclear energy and has significantly stymied the advancement of energy generation in ways that cannot be fully understood. And, although nuclear is the worst example of overregulation, just about any highly regulated industry can demonstrate just how much it costs to compete in the market, often times to the detriment of the industry.
Treating AI like a highly regulated industry will set the United States back decades. In doing so, it will create an environment that only the largest companies will be competitive in (because only they can afford it) and will only further restrict the innovations that will be brought to market. Further, the downstream industries where AI can and is having an impact today, for example: medicine, healthcare, and energy already have their own regulations that will have an impact on the application and use of AI in those spaces. To regulate AI at a general level would effectively double tax and double regulate the technologies that these sectors are already finding use for.
Regulation can spur economic growth by focusing on people
We should create an approach to regulation that doesn’t focus on the AI product itself, but instead focuses on creating an empowered workforce that is trained and equipped with the appropriate ethics, credentials, and frameworks to supercharge AI in our society. We can look at mechanisms around medical practice, engineering, legal, and even cybersecurity on how to create a professional workforce that will have the right education, ethical grounding, and government backing to make the right decisions for both the science and business.
A national educational framework and institutional credentialing
Anyone who has followed the National Institute of Standards and Technology (NIST), the Cybersecurity and Infrastructure Security Agency (CISA), and the National Security Agency (NSA)’s efforts to bolster our nation’s cybersecurity workforce can’t help but be amazed at the effectiveness of their programs. From NIST’s National Initiative for Cybersecurity Education (NICE) educational framework to the CISA NSA National Centers of Academic Excellence – Cybersecurity, there is now a robust and consistent educational story around cybersecurity practitioners.
AI should have a similar educational story, driven by the National Science Foundation (NSF) with help from NIST. Something like a National Center of Academic Excellence in Artificial Intelligence could play a critical role in executing an effective national strategy and would be a strong complementary partner to the National AI Initiative. Creating a strong ethics focus is a cohesive effort that crosses many organizations and impacts many of the suggestions here. There is already a significant focus on ethics and impact from government organizations like the Chief Digital and AI Office in the Department of Defense and other civilian agencies.
Part of a credentialing structure should be a strong focus on ethics and the humanitarian implications of AI. This approach, implemented effectively, guarantees a robust workforce. A credentialing structure would also use learning from the many years of work wayfinding for cybersecurity and can be executed far more quickly for this new domain.
Personal accreditation is also community accountability
The other half of a proper regulatory framework should focus on people, not a product, and borrow from other professions that follow similar accreditation efforts as legal, engineering, and medical fields. To start, a single national registry of practicing AI professionals, hopefully with strong ties to a National Center of Academic Execellence in AI (NCAE-AI), would support other national efforts. And in fact, the use of technology could make this effectively a nearly double-blind system providing attribution without identification.
Personal accreditation along with a digital component would provide a critical piece of the puzzle that mirrors other professions. A lead researcher or commercial scientist would attest a model in the same way that a P.E. attests to a bridge or an M.D. attests to a procedure or practice. It puts the power of the trade into the hands of an individual, reinforced through the accrediting institutions.
Personal accountability of machine models, like other professions, puts the power and responsibility of the impact on society into the hands of the implementer – exactly where it should be. Accordingly, it also functionally accommodates tort law, and the ability for corporate and government entities to largely escape legal challenges unscathed. In technical practicalities, large AI projects will require attestation by many researchers, whereas smaller start-ups and research and development teams who may only have a single professional can still be adequately represented without the onerous requirements of lengthy audit delays and governmental red tape.
Mechanisms like this only work when the community is actively protected, however. This next part takes lessons from whistle-blowing laws and medical practice reviews.
The reality is that unscrupulous people can exist anywhere. One of the best mechanisms to avoid this sort of intentional malfeasance or breech of the ethics codes, formalized by a NCAE-AI, simply involves the ability to directly whistle-blow on these breeches. This is where government reactive involvement makes more sense. Whistle-blowers would need to be protected, and legislation would need to support both investigations, adjudications, and any civil or criminal penalties.
In addition, to directly attribute models to an individual, the community should also actively participate in regional and national conferences that take on the role of reviews and learning sessions. This is already done in many academic circles, and the key would be to formalize it alongside the institutional accreditation frameworks.
AI regulation doesn’t have to be hard
These suggestions, brought forward from existing constructs already provide value to their respective industries, and are an effective way to create strong guardrails and frameworks to support the appropriate use of AI in society without endangering the technology of over regulation and extinguishing a barely lit flame, or worse consolidating the power of a tremendous technology in the hands of a techno-oligarchy. The strategies introduced provide a credible alternative option to direct regulation, reinforced by centuries of good practice and societal advancement.
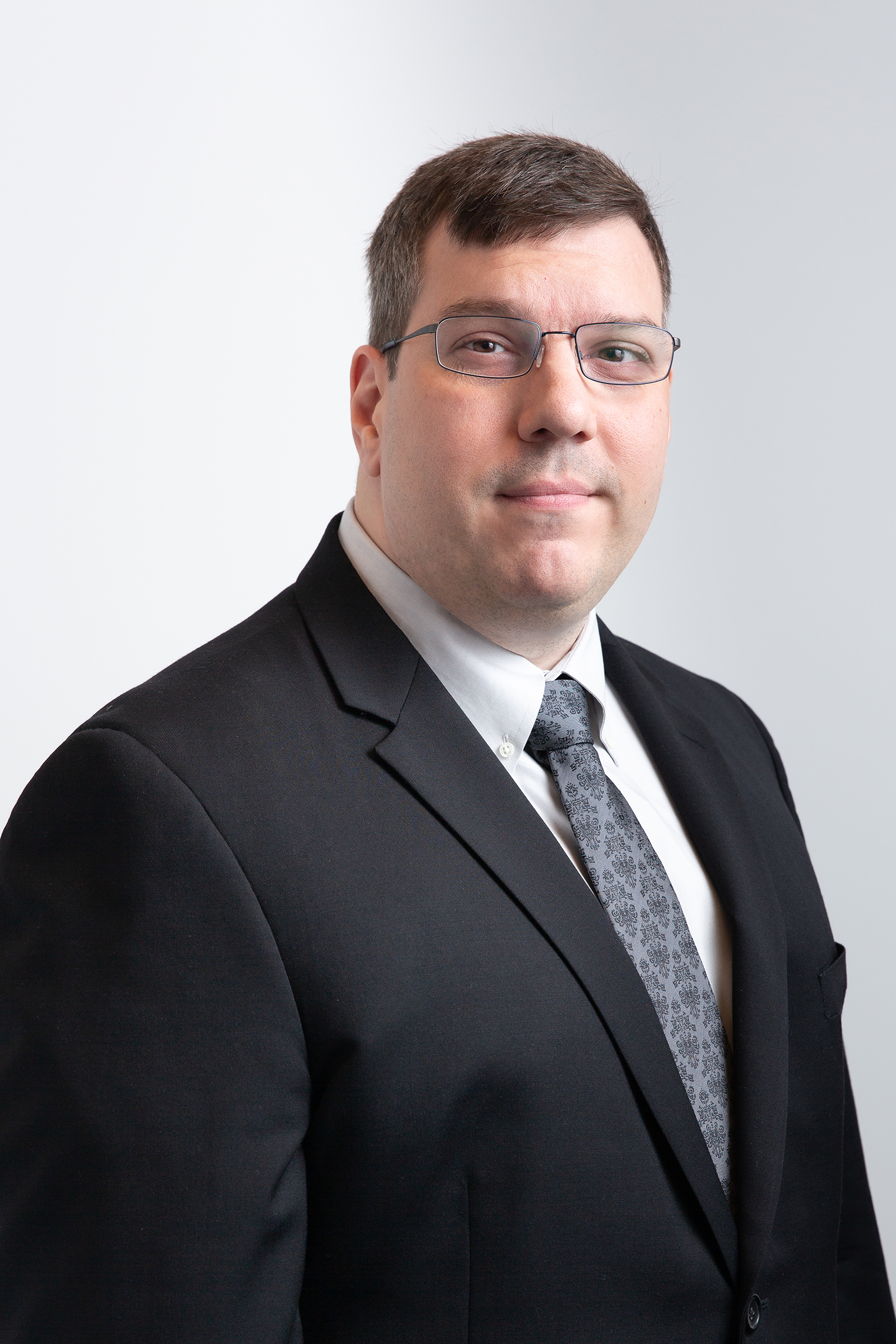
John Janek
Chief Technologist