AI is coming for your job
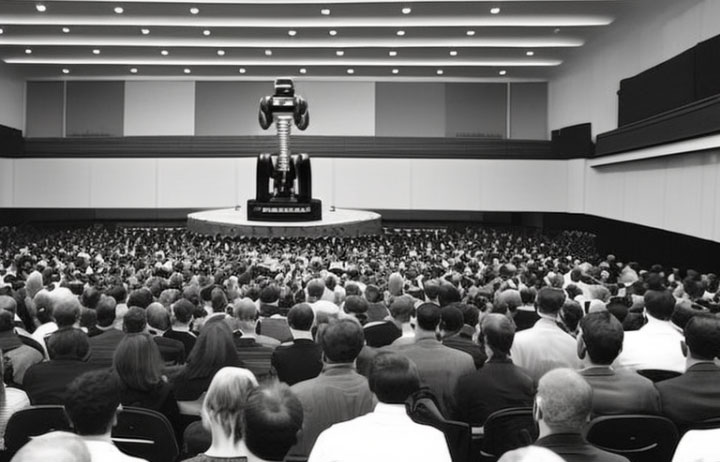


If software ate the world, AI is remaking it.
This is a story about being human, and the importance of that trait in a future of ubiquitous technology. It is a recap of a discussion with Miami University Emerging Technology in Business + Design students on 4/27/2023 on how to think about personal positioning in the tech workforce of the future.
The Highlight Reel
If you’re graduating soon, things are changing. If you’re graduating in two or three years, things will have already changed by the time you’re done with your program. That isn’t a fault of anyone in particular. When you hit a point where disruptive technology becomes functionally ubiquitous, there are all sorts of crazy things that start happening. Sometimes new opportunities and even new markets emerge, and sometimes you wind up feeling out of position and with the wrong toolbox for a remarkably unforgiving job market as entire traditional sectors contort and, in some cases, disappear completely. We’re going to cover the state of play in the AI space at this particular moment in time, a bit about how to think about this moment in context of delivering value, and then I’m going to end with some things to think about moving forward.
Let’s start with a baseline. Artificial Intelligence isn’t new. It’s all around us, driving interactions every single moment of the day. It already has a profound impact on many industries and use cases in nearly every commercial, non-profit, and governmental context. It has caused controversy. It has caused serious reflection and introspection on how data can bias and exclude people. It has created discussions around how better to interact with technology.
Like other disruptive technologies before it, we see a lot of similar indicators. That’s what I’m here to talk about today. Not the outliers – computers are very good at seeing those – but what’s human, and what’s similar to what we’ve seen before. The human part is key: this talk should be delivered without technology, as a conversation between people. I’ll come back to that later.
The emergence of AI has been a multi-decades long process. The math that forms the foundation of most computational science goes back literally decades, sometimes even half a century or more, and modern approaches to synthetic learning nearly half as long. This tendency is exactly the same regardless of what technology you’re looking at. There is usually a long arching epoch of research, study, investigation, and development that led to this point. No, Elon Musk did not just wake up one day hungover and say that cars should drive themselves. Nor did Steve Jobs stumble on his way to the office one morning while fumbling with Blackberry and ideate his way to an iPhone.
So what’s different? A catalytic event or turning point suddenly made the technology approachable. I use the word catalytic because it’s energetic. It’s transformative. For the iPhone, it was a handful of technologies brought together in an innovative way that hit a particular price point. For Tesla it was the same thing to some extent: the right technology at the right time for the right people. Yes, this is over-simplification. Regardless, the impact of critical technologies hitting a maturity and ubiquity to be brought together in new ways cannot be underestimated.
The biggest change has been in the way that AIs now are interacting with the market and comes with the explosion of large language models (LLMs). These models are specifically designed and trained to perform in manners that look and feel “human”. Make no mistake: they are not. It’s a predictive algorithm based on an incredible amount of training data – most of it from the Internet (that alone should tell you something). They are, however, an effective facsimile and useful for moving data between humans and computers. In this regard, they provide us with an unstructured human machine interface (HMI) that looks and feels natural.
Led by this emergence of LLMs, the catalyst that makes now possible is access to compute. The math behind artificial intelligence is easy to see, easy to experience, and mind bogglingly complex at scale. You can learn the basics with YouTube and Excel. But you need hardware – in some cases very expensive parallel computational architectures – in order to really explore the space. You also need access to incredible amounts of data against which to run these algorithms. And for the last two decades or so, we’ve been building those infrastructures out through a combination of advances in networking, data centers, and parallel and high-performance computing.
No human or team of humans, regardless of how large, would ever really be effective at it. It happens at a scale that is nearly impossible to conceive of. For example, the generative AI called stable-diffusion by Stability AI has a checkpoint with more than 850 million weights. Try solving an equation like that in your lunch hour. And yet, they’ve managed to develop models that can predictably create amazing, human-recognizable images in moments on consumer hardware. That’s what a catalytic moment looks like.
Utility, Value Streams, and the Ubiquitous Tech principle
With that understanding that we have reached that critical tipping point that AI will become disruptive eminently, I feel it’s a good time to transition into the economics of it. I want to focus on a couple of high-level items here: utility, value streams, and what I like to call the ubiquitous tech principle. This won’t be a definitional discussion, for each of these topics there are plenty of very good resources to study. Instead, I want to focus on why these terms matter in the context of finding human or social value.

Let’s start with utility.
We’re going with the basic economic definition here that utility is the ability to define the worth or value of a particular good or service. As with most things in economics, the terms are incredibly subjective, and as the world continues to increase in complexity so too are the more traditional ways of assessing worth or value. For example, the most common way we tend to explain this is through the transaction that can occur to attain the thing in question. If we spend a lot on it (maybe even many times what the cost of product is), then it is valuable. That is a pure market perspective, however, and doesn’t take into account many of the very complicated social and human aspects that have been accelerated, perhaps exacerbated by the Internet. Today the realized utility of something can be quite different than the average utility of the transaction, driven mainly by the individual preferences that tend to be asserted throughout the process.
Many economists would tell you that this is accommodated in the models and averaged out. I would suggest that as technology increases in ubiquity and drives toward commodity the actual range of utility for any given transaction also increases by a substantial amount driven by a wider range of consumers in the space. That’s a complicated way of saying that value needs to be far more specific to the individual, and this is driven by the ubiquity of a good or service.
On value streams.
With the idea of individual utility in mind, let’s transition to a talk about value streams. Again, just for a baseline the value stream is the flow of activity, usually both information and material, between a creator and a consumer. If you make bikes, your main value stream is the process of assembling those kits to deliver to people all over the world. It comes from the Lean Six Sigma world, which adheres to those core Lean/Kaizan concepts, including efficiency through the elimination of waste.
Any particular value stream tends to be very focused on creator and consumer models, which is typically an approach used to control scope and control over a process for improvement. When you string a bunch of value streams together, they become a value chain. And that’s all fine and well, but it tends to disaggregate a value stream if there isn’t an experienced and user/human-centered approach to the work. In the example above, there might be many value streams in the process of delivering a bike kit to a home. At the end of the day, however, if the consumer doesn’t know about the bike, can’t order the bike, can’t receive the bike, and can’t assemble the bike there isn’t much value to be had.
The Ubiquitous Technology Principle.
It is an imperative that any value stream always be considered in the context of the ultimate consumer outside of the organization. This creates context, outcome-orientation, and a sense of place and purpose. It is even more critically important for organizations who consider their primary value streams to be other “customers” inside the organization. When done consistently, this approach is one of the best methods to removing organizational silos and barriers to collaboration.
As technology approaches true ubiquity – as in there is little to no energy expended to deploy it into a value stream – there is a diminishing return around human-instigated lean approaches. Basically, the technology will reach a point where it effectively replaces all people in the value chain. I call this the Ubiquitous Technology Principle.
Being Human is the One Thing AI Can’t Replace
If you’ve come with me this far, that’s great. If you’re reading this and TL;DR’d down to here, welcome.
If the purpose of ubiquitous technology is to replace any person in a value stream or chain, we are left with an uncomfortable question. What happens to the people? This is why there are so many technologists who are actively against the use and adoption of AI. Because they see the ubiquitous technology as a threat, not an opportunity.

I want to offer a different perspective. If ubiquitous technology offers a theoretical perfect implementation of a value stream, then there are still two roles which are critically important: the creator and the consumer. We’ve seen over the past two decades how important the role of being human is in our digital interactions, and we’ve seen the harm that can happen when we either don’t understand or truly reflect on the value streams that we interact with. We see this consistently today in the premium value associated with truly human-to-human exchanges. Dealing with a person is possibly the least efficient way to accomplish a value stream. But it may ultimately be the most effective for that critically subjective concept of utility.
Now, perfect value streams are years, decades, possibly even centuries away. But if history has taught us anything, it is that the concept of ubiquitous technology and the impact it plays in reducing the human role in value stream delivery cannot be stopped. I believe that we are approaching a cross-over point where because technology is so ubiquitous that technology stops being a differentiator. Instead, it becomes a differentiator to implement these human and people-centric approaches and to focus on the creator and consumer. We already see this in premium or luxury use cases, and in a world where ubiquitous technology has driven out all the waste in a value chain, the only difference will remain in the people who act on behalf of the creators and suppliers.
The Attributes of the Future
As a professional in this brave new world, how can you best position yourself? What are the things that you need to be aware of, hone, and use on a constant basis in order to be the human-centered creator that will continue to drive demand in the coming years. There are some core attributes that I want to share with you.
Curiosity – this isn’t just being nosy. Curiosity is a challenge to ask yourself five times as many questions as you make in statements every day. It is to look at processes and workflows and wonder how they impact or are connected to someone outside the organization. It is the skill to ask questions that seek understanding, that drive clarification, push for action, and most importantly that encourage others to ask questions and understanding, too. Curiosity in a world of ubiquitous technology is the fundamental attribute that will continue to drive innovation, ensure active participation, and a role in a human-centered workforce.
Caring – this is probably going to be spicier. I talked about this as commitment in my original talk, but caring really does encompass the diverse spectrum of action that is involved. Caring is commitment, empathy, and collaboration all rolled into one. It’s showing up for the work even when you don’t think anyone will notice. It’s about doing the right thing when it isn’t the easy thing to do. It’s about being honest with yourself and those around you. To end on a cliche, it’s putting the shopping cart back in the corral.
These attributes, like any other, must be practiced and trained in order to be honed. There is always something new to learn, some new perspective to understand, and someone’s opinion to value. And, most importantly, even if you’re not a naturally curious person, these are attributes that can be enhanced through conscious, consistent, and persistent application. They will always be things that anyone can fall back on in order to understand what their next step looks like.
And the Skills Needed Today

If you’re in the tech workforce today, there are a couple of skills that you need to keep in your back pocket for the next 12-18 months.
Data Engineering – One skill that is often overlooked is actually a group of skills I like to call data engineering. These skills have always been important. Storing, retrieving, modifying, and using data has always been critical as the software we use has become more complex and more complicated. Often, we sacrifice good practices around data engineering for speed, and in the context of AI this can be a costly mistake. Data is the fuel that AI uses, and it has to be refined to be usable. Learning how to perform those core data engineering tasks, with an eye for the sorts of data structures and meta data that are most useful for model development, can set you apart in meaningful ways.
Prompt Engineering – Going to stick with the spicier takes. Prompt engineering wasn’t on anyone’s radar a year ago. In the intervening time, it has become a discipline that many are arguring won’t last long. Sam Altman over at OpenAI has already said that the era of LLM competition has come and gone. But that doesn’t mean that LLMs are going away. Companies will find ways to use them better, to add better user interfaces and user experiences, but the core of the general-purpose nature of the LLM means it will be part of the toolbox for a long time to come. If you’re going to be in tech, then you better know how to use those general-purpose tools. Prompt engineering is a great way to leverage core tools in the same way that knowing Bash, Python, or Docker can help with many day-to-day engineering problems, or SAS, SPSS, or MatLab might help you with a data science challenge. Will it stick around as a discipline? That’s a bigger question. For now, it certainly is a very useful skill to have.
There’s an apocryphal quote out there: “In the future there will be people who learn to work with AI, and people who are unemployed.” There’s a lot to unpack in that statement, and I believe quite a lot of truth there too.
So all that was a really long way to say…
Technology continues to change the future, as it always has. We’re at an epoch moment, where the implementation of these AI tools is going to be disruptive, and will continue to disrupt delivery in the foreseeable future. That doesn’t mean you should be afraid, and it doesn’t mean you should hide. Instead, leverage the unique traits that make you, you. Find ways to delivery value in truly human ways, and for the foreseeable future you can’t go wrong. And in the meanwhile, learn the skills that allow you to leverage these new tools to deliver value better, more effectively.
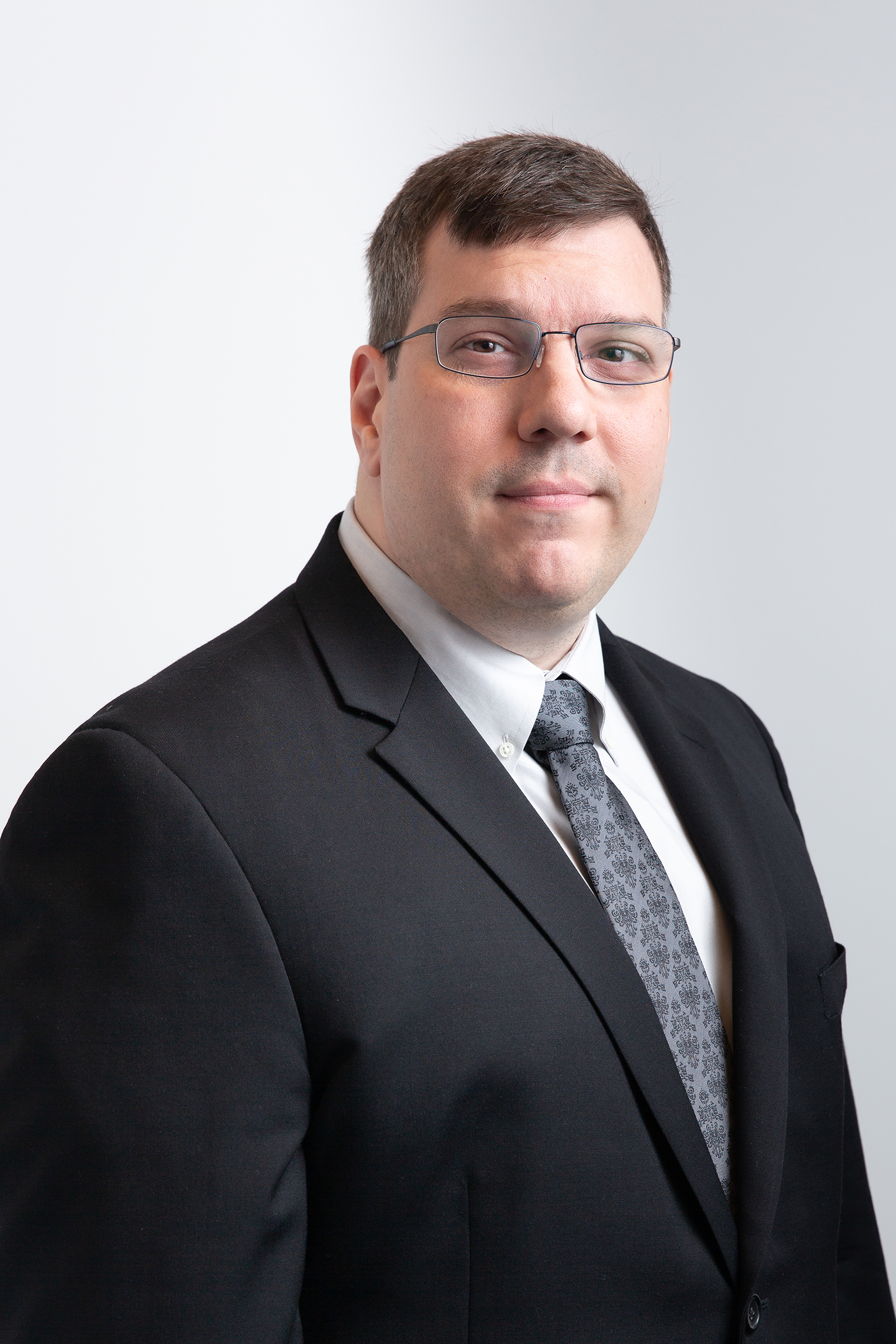
John Janek
Chief Technologist