An AI Looks At Human Writings On Ethical Artificial Intelligence
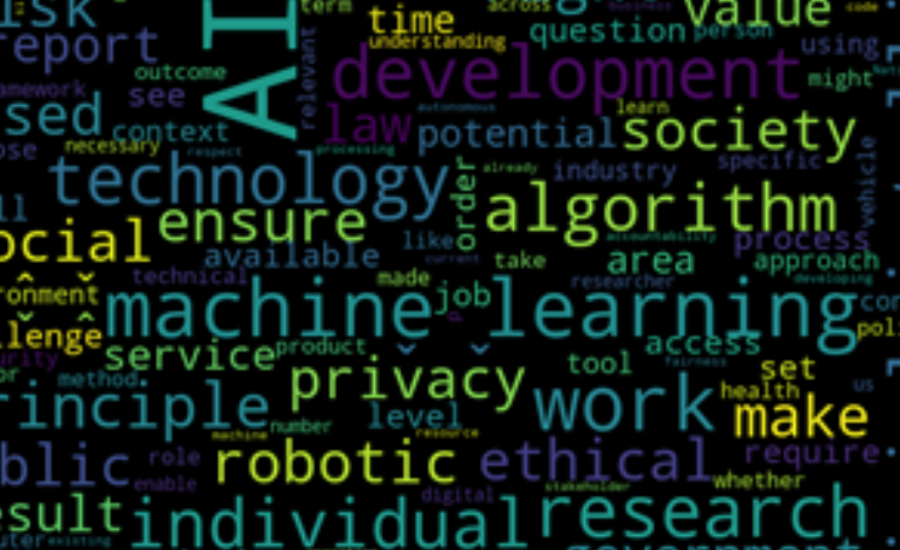


Applications of artificial intelligence (AI) have affected business, society and governments in significant ways. Many benefits have been realized, including better access to information, improved health outcomes and more efficient business processes. These advances have not come without drawbacks and risks, however. AI’s impact on employment, personal freedoms and the environment have been disruptive, and AI in the hands of bad actors will certainly pose new threats. The past three to five years have seen a call for an ethical approach to AI that addresses its potential negative impacts, mostly through the publication of dozens of guidelines, declarations, and frameworks for ethical AI. At Dev Technology, we have decided to adopt the Joint Artificial Intelligence Center’s (JAIC) AI Ethical Principles: Responsible, Equitable, Traceable, Reliable and Governable. Nevertheless, we think it is important to understand the concerns and recommendations across the many national, international and non-governmental organizations (NGO) who have published work in this area.
Approach
We picked 90 recent documents which addressed the topic of ethical AI in some depth. This represents about 2,000 pages of text content—too much for a human to thoroughly digest, so we applied some of the very algorithms which were the subject of their contents. We wanted to understand the major themes developed within these documents, the more specific vocabulary of AI ethics, and what steps these organizations recommend to AI practitioners and consumers. We of course considered the ethical aspects of what we practiced in this exercise. We used these algorithms to make this data more transparent and readily perusable. We modeled exclusively the text present in dataset itself – we did not re-use a model trained on some other, potentially biased, corpus and then apply it here.
The algorithms we used include tokenization of natural language text; noun phrase extraction; TF-IDF ranking of vocabulary; mutual information analysis; principal component analysis (PCA); and k-nearest neighbor similarity ranking (kNN). These tools helped us quickly find the major themes that ran through the contents that we selected. They helped us organize the documents, as well as develop a topical vocabulary that could serve as a kind of unified table of contents or back-of-book index.
Findings
Not surprisingly, the most prevalent theme, developed seriously by 85% of the documents, was centered around ethics. This in itself is not a very interesting observation – we also wanted to know how this theme was addressed, and what specific vocabulary was chosen to develop the idea. There were more than a thousand different phrases developing the concept of ethics, including some that the AI resolved as particularly important: ‘ethical boundaries,’ ‘ethical implications,’ and ‘ethics committee.’ This last phrase interested us, and we dove deeper to find that many of the publications called for ethical oversight committees within both practitioner organizations and customers of AI.
The AI found that the topic of bias, a clear hot button in current AI news, is addressed in 70% of the documents. Specific sub-topics within this domain included ‘biased models,’ ‘uncritical bias,’ and ‘preventing discrimination.’ The Humanitarian Data Science and Ethics Group (DSGE), for instance, published a Framework for the Ethical Use of Advanced Data Science Methods in the Humanitarian Sector, for which issues of bias and fairness are significant concerns.
Autonomy was another major theme throughout, addressed by 70% of the publications. The AI, somewhat surprisingly, found a smaller vocabulary describing the topic, about a third as diverse as the vocabulary of ethics. Some important themes were ‘autonomous weapons,’ ‘verifiable autonomy,’ and ‘agent-based autonomy.’ The related topic of robotics showed up in 55% of the documents. One sub-topic was centered around ‘robot personhood.’ In fact, although we usually think of AI ethics in relation to the effects of AI on humans, in the robotics domain there is a reasonably-sized discussion about the point at which humans will have to recognize the rights of artificial constructs.
The effects of AI on employment was another theme the AI found, discussed in 60% of the documents. Important trains of thought were ‘labor policy,’ ‘STEM jobs,’ and ‘employment outcomes.’ The eventual outcome of being able to automate many repetitive tasks, even those of knowledge workers, is not easily predictable. However, without serious policy attention, the path from where we are now to that end state will be a rocky one.
Applications of AI to the healthcare domain was one of the easiest topical groups for the AI to identify and highlight. 70% of the documents had something to say about it, and several of them were dedicated only to this vertical slice of applied AI. ‘Health diagnoses,’ ‘healthcare expenditure,’ and ‘medical mistakes’ were all important themes within this discussion. Medicine is a field in which there is already a long tradition and large body of work devoted to the ethical responsibilities of practitioners. The Academy of Medical Royal Colleges could consult numerous medical and bio-ethics experts when they published their Artificial Intelligence in Healthcare Report.
Conclusions
Thus far, we have been looking at the descriptive modeling of this collection of text, powered by a language-aware AI, but fairly neutral in application. What any discussion of ethics in a largely unregulated domain such as AI/ML needs is a prescriptive model – what should we be doing? What, on the whole, were some of the most prevalent mitigations that the organizations who published these documents foresaw could make the creation and application of AI subject to human ethical norms? We have highlighted some of the specific recommendations from documents which the AI saw as particularly salient with respect to recommended changes to existing practices:
- The Future of Computing Academy recommends a change to the peer review process for new AI research which would emphasize the reasonably likely broader impacts of the research, both positive and negative.
- The Asimolar AI Principles, 23 short statements on a variety of ethical principles for researchers and practitioners, is widely cited but lacks definitions that would allow an organization to easily implement them specifically.
- Working from these basic principles, the World Economic Forum published a white paper titled “How to Prevent Discriminatory Outcomes in Machine Learning” which lays out specific steps to implement Active Inclusion, Fairness, Right to Understanding and Access to Redress. Many of these steps are already practiced in finance, medicine and information security, including the establishment of standards bodies, periodic audits and enforcement-empowered governance structures.
The authors of these documents seem to feel that human constructs for ethical practices already exist, and simply need to be adopted by research and industry communities that have so far stayed outside those formal structures. It is an open question whether the very nature of these technologies makes them incompatible with regulatory processes that are executed manually and operate on the scale of months and years.
Joshua Powers
Technical Director of AI & Machine Learning
Dev Technology